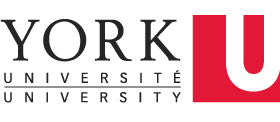
Collaborating with a team from Sunnybrook and U of T, a grad student in the Lassonde School of Engineering leverages artificial intelligence – machine learning – to develop a new tool for symptom management in the breast radiation oncology clinic. It could have wide application in cancer treatment.
(View on York University’s website)
New research from York University represents a remarkable step forward in personalized breast cancer treatment. Lassonde School of Engineering PhD student Khadijeh Saednia, in collaboration with Sunnybrook Health Sciences Centre, the University of Toronto and others, investigated a novel application of machine learning to detect skin toxicity (or damage) from breast radiotherapy much earlier than was previously possible.
This study proved the feasibility of artificial intelligence (AI)-assisted image-guided approaches – specifically, Quantitative Thermal Imaging (QTI) – as a new clinical decision support tool for symptom management in the breast radiation oncology clinic.
This was possible through earlier detection using machine learning methodologies: “Patients undergoing radiation therapy, or RT, would benefit from earlier detection of skin damage or toxicity because symptom management could be introduced sooner than is the existing practice. These individuals could experience an improved quality of life during and beyond treatment,” Saednia emphasizes.
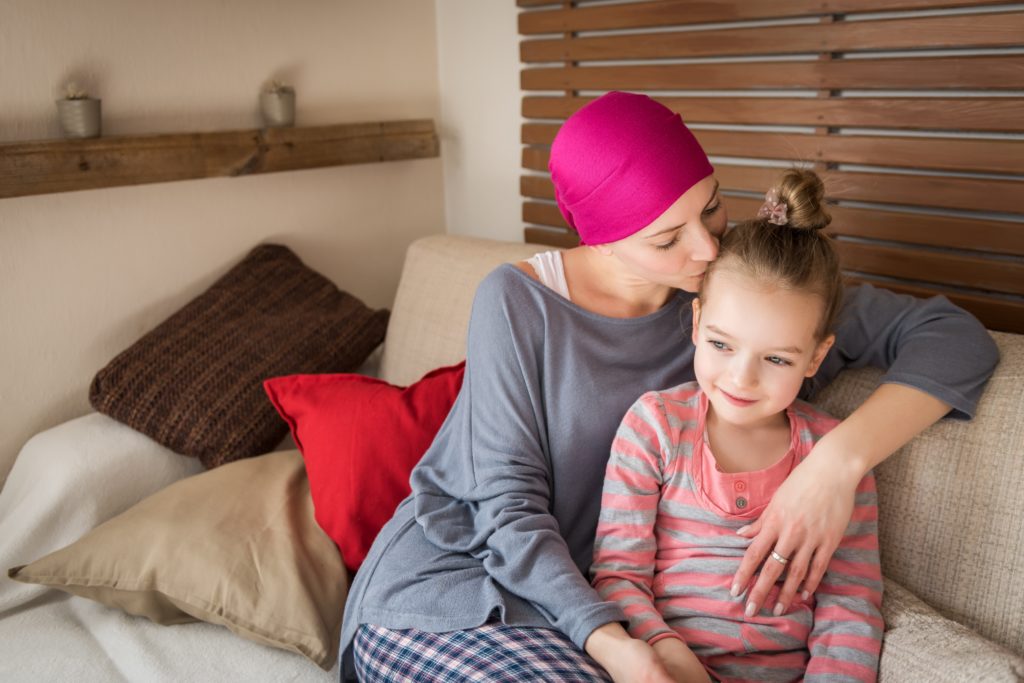
This original research was supervised by Lassonde Professor Ali Sadeghi-Naini, York Research Chair in Quantitative Imaging and Smart Biomarkers, and Sunnybrook Scientist Dr. William Tran, funded by the Terry Fox Foundation and published in the International Journal of Radiation Oncology*Biology*Physics (2020).
Radiation therapy, a key part of post-operative management, often has side effects on the skin
This research fills an important void. Breast cancer is the most common cancer among Canadian women (excluding non-melanoma skin cancers). It is the second leading cause of death from cancer in Canadian women. It is estimated that, in 2020, 27,400 women will be diagnosed with breast cancer – that’s 25 per cent of all new cancer cases in women in 2020. (Canadian Cancer Society)
RT, which uses ionizing radiation to target residual cancer cells of the breast, is a crucial component in the postoperative management of breast cancer. But the side effects from this treatment may affect patients’ quality of life since the skin is susceptible to radiation damage and toxicity. This can mean pain and discomfort for these patients. That’s one of the reasons why patients undergoing RT are carefully monitored.
From the left: Ali Sadeghi-Naini and Khadijeh Saednia
Saednia, who specializes in AI and machine learning for cancer management, turned her attention to one common side effect of RT in breast cancer patients: dermatitis. She suspected that thermal imaging in conjunction with machine learning could help because it could detect the damage earlier than previously possible.
She explains how this would work: “Physiological changes associated with radiation-induced dermatitis, such as inflammation, may also increase body-surface temperature, which can be detected by thermal imaging.” Quantitative imaging techniques coupled with machine learning can potentially be adapted to detect such alterations earlier after the start of RT.
So, she investigated the use of QTI biomarkers and machine learning for early detection of radiation-induced skin toxicity in breast cancer.
Ninety patients recruited from Sunnybrook
Saednia’s study took place at the Odette Cancer Centre at Sunnybrook Health Sciences Centre, Toronto. Image reproduced with permission of Sunnybrook.
The research team recruited 90 patients who were being treated for RT. The study took place in the Department of Radiation Oncology at the Odette Cancer Centre at Sunnybrook Health Sciences Centre in Toronto.
Thermal images of the treated areas of these patients were acquired at various intervals: before RT, then weekly. Parametric thermograms, which measure heat, were applied and their findings analyzed. The thermograms were used to derive quantitative thermal-based features that included surface temperature and texture parameters. Skin toxicity or damage was evaluated at the end of RT using the Common Terminology Criteria for Adverse Events guidelines.
Results: Researchers were able to predict skin toxicity much earlier
Thirty-seven patients, of the 90 in the study, exhibited adverse skin effects, and had significantly higher local increases in skin temperature, reaching above 36 C.
The timing of this key finding is what’s important; the researchers’ ability to measure skin toxicity earlier than previously possible is key. Skin toxicity is typically observed after the 10th RT session (or fraction) or after 10 or 14 days of initiating RT. Instead, Saednia and her team, using QTI with machine learning, obtained this information at the fifth RT session, demonstrating early prediction capabilities to severe skin toxicity.
“Machine learning models demonstrated early thermal signals associated with skin-toxicity after the fifth radiotherapy fraction with high prediction accuracy,” she explains.
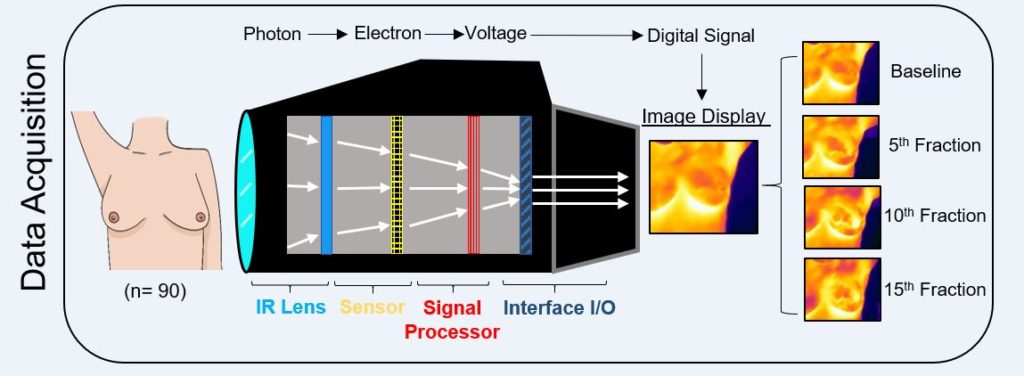
“Our study concluded that QTI can be used to detect changes associated with radiation-induced dermatitis and can be integrated with machine learning frameworks to develop a predictive tool for skin-toxicity assessment at early treatment times,” adds Sadeghi-Naini.
Saednia is confident that a significant patient population would potentially benefit from early detection and early intervention for symptom management. She emphasizes the direct application of this research: “We propose that ‘smart’ QTI be used as a clinical tool in radiation oncology.”
To read the article, “Quantitative Thermal Imaging Biomarkers to Detect Acute Skin Toxicity from Breast Radiotherapy Using Supervised Machine Learning,” visit the publisher’s website. To learn more about Ali Sadeghi-Naini, visit his Faculty profile page.
To learn more about Research & Innovation at York, follow us at @YUResearch; watch our new video, which profiles current research strengths and areas of opportunity, such as Artificial Intelligence and Indigenous futurities; and see the snapshot infographic, a glimpse of the year’s successes.
By Megan Mueller, senior manager, Research Communications, Office of the Vice-President Research & Innovation, York University, muellerm@yorku.ca